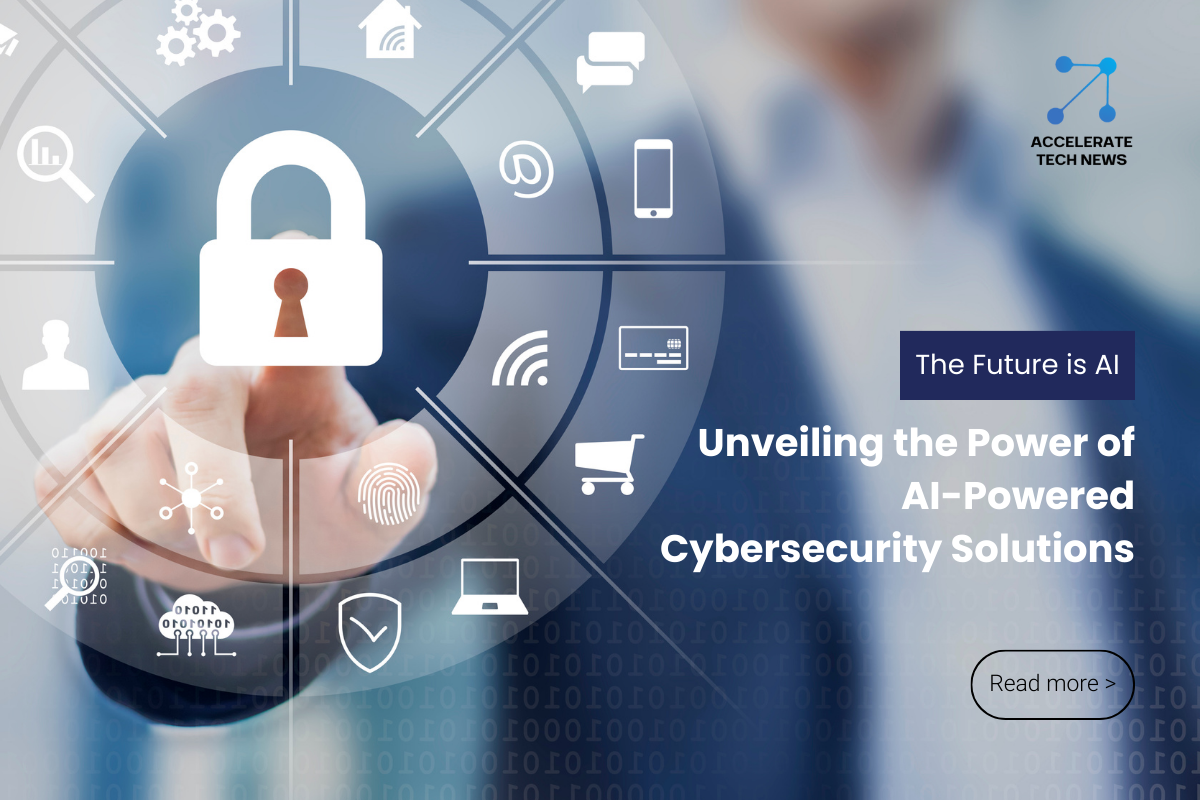
The volatility of the cyber terrain necessitates organizations to adopt the power of innovation to reinforce their cyber defense systems. AI-based cybersecurity systems are fortunately emerging as immutable and effective warriors in the fight against cyber criminals.
Did you know that 88% of these large companies came under different spear-phishing attacks in the last year? And that the average cost of a data breach reached $4.35 million in the year 2022? This disturbing data makes us realize that the development of solid cybersecurity tools is an urgent necessity in today’s day and age.
I. Understanding AI-Powered Cybersecurity Solutions
A. Machine Learning in Cybersecurity
The essence of AI-based cybersecurity solutions is hidden within machine learning algorithms. With the help of these highly efficient systems, data processing becomes much more efficient, and analysts can identify even the most subtle patterns and trends. Machine learning can think ahead of the evolving cyber threat and be able to counter the enemy’s move in every way.
B. Threat Detection and Prevention
AI-driven cybersecurity solutions are demonstrating their worth in many aspects of the cybersecurity field, such as finding and stopping malware and ransomware attacks, phishing scams, and advanced persistent threats (APTs). By making use of real-time threat intelligence as well as proactive defense mechanisms, these cybersecurity solutions can identify and neutralize threats thus preventing them from causing major damage.
C. Data Protection and Privacy
The digital world makes data a valuable asset, and AI is one of the key actors in improving data security procedures. AI-driven solutions are the newest in modern-day armor, providing advanced encryption and anomaly detection, as well as granular access control that are the final shields around the sensitive information of businesses. These AI-driven solutions ensure that a business can defend its assets with the utmost vigilance.
D. Behavioral Analysis and User Monitoring
AI algorithms are not only capable of sensing the outer threats but also are good at detecting the inner user behavior which may lead to the insider threats. Through ongoing monitoring and analysis of user activities, AI-enabled solutions can detect even the slightest sign of danger and take adequate actions. Insider misconduct has been reported to be the cause of 23% of data breaches that occurred in 2022, per a latest report.
E. Network Security and AI
Network security has always been the key element of complex cybersecurity strategies, and now AI is changing the game in this field. AI-powered algorithms can analyze network traffic in real-time, detect security breaches, and prevent unauthorized access, virtually protecting businesses from cyber-attacks.
II. Implementation and Challenges
A. Implementing AI-Powered Cybersecurity Solutions
Implementing AI into the existing cybersecurity framework can be a complex and complicated task. Organizations need to investigate their existing system in detail, identify the potential issues in terms of compatibility, and develop a plan to integrate the new system smoothly without any disruption to critical security operations.
Data readiness is also an important element in the successful implementation of the platform. AI algorithms are data-hungry and need lots of clean data with labels to be trained properly. Yet, most organizations have a problem with the data, which is scattered and fragmented, and this can be the reason behind the poor performance of the AI-powered systems. Implementing stringent data governance models and dedicating funds to data collection, preprocessing and management are vital.
Moreover, agencies should endeavor to improve the skills deficit by offering comprehensive training to their cybersecurity teams. AI-driven solutions necessitate the creation of dedicated teams of data scientists, machine learning experts, and AI model creators and deployers. The lack of investment in upskilling and hiring the appropriate staff may worsen their efficiency.
B. Challenges and Limitations
Data Quality and Availability: The AI accuracy is as good as the data they are trained on. Inadequate, incomplete, or biased data can generate inaccurate predictions and suboptimal performance of AI cybersecurity solutions, which will result in false positives and false negatives. Access to excellent, diverse, and unbiased data is a continuous problem.
Computational Resources: AI model training and implementation is a process that is quite demanding computationally, in terms of processing power, memory and storage resources. Such a situation is usually faced by small and medium-sized enterprises in their limited IT budgets, which can be a problem for them as they may not be able to afford advanced AI-powered solutions.
Adversarial Attacks: As AI grows in cybersecurity, the ways of bypassing or manipulating AI models, known as adversarial attacks, may become the tool of malicious actors. These assaults can encourage the AI systems to make a false positive of threats or make inaccurate decisions, which will result in the systems not being effective enough.
Bias and Fairness: AI algorithms may develop biases present in the training data or the modeling process, thus potentially leading to undesirable fairness or discrimination. The need to maintain equity, accountability, and transparency in AI-based cybersecurity solutions conscientiously is a serious concern that must be addressed consistently.
Interpretability and Explainability: The great proportion of artificial intelligence models, especially deep learning architectures, are called “black boxes”, thus the explanation of their decision-making processes is a problem. This leads to the AI-based solutions being accepted less as they are not interpretable and explainable which raises the fears of compliance and accountability.
Human Oversight and Trust: In contrast to AI which can handle most cybersecurity tasks and be a support, human involvement and supervision remain the most important factor. AI systems may sometimes make errors or come upon unpredicted cases that demand human intervention and decision-making. Trust in AI solutions which are technologically more advanced, and concrete rules and regulations for their implementation are the key factors to commercial and operational success.
A comprehensive approach involving technological breakthroughs, robust data management, specific skills development, and major ethical AI principles is needed to tackle these challenges and limitations.
III. Future Trends and Innovations
The AI security sector is changing rapidly, and there are new breakthroughs and inventions every single day. The future may open amazing horizons for those who are brave enough to accept these latest trends in technology, just like a superhero who is always in search of the latest gadgets and innovations to upgrade his abilities.
Reinforcement Learning and Adversarial Machine Learning:
Reinforcement learning, a branch of machine learning inspired by behaviorist psychology, enables AI systems to keep learning from the past and continuously adapt their play styles. It can be ahead of the game predicting and intercepting cyber threats as it happens in real-time.
Adversarial machine learning, on the other hand, is the process of training AI models to be resilient against attacks from adversarial sources, during which it attempts to trick or deceive the system. Integrating adversarial training technique into the training process can be used to make the cybersecurity solutions more resilient and ready for AI attacks that make systems vulnerable to attacks.
Quantum Computing and Cybersecurity:
The arrival of quantum computing leads to both challenges and opportunities for cybersecurity. On the other hand, quantum computing can lead to the creation of totally un-hackable encryption algorithms, which will allow for the highest security level of data. On the one hand, it puts current cryptographic protocols in jeopardy as a quantum computer can possibly crack many of the existing encryption standards. To overcome this, scientists are now busy looking into quantum-safe cryptography and post-quantum cryptography solutions. These approaches focus on creating cryptographic algorithms that are robust against both classical and quantum computers to facilitate the sustained security of digital communications and data.
Blockchain-Based Security Solutions:
Blockchain technology, which is famous for its decentralized and transparent nature, helps for various cybersecurity purposes. Through blockchain-based solutions, it is possible to enhance the integrity of the data and enable secure information sharing at the same time, while keeping a tamper-proof log of security events. A prospective use of blockchain here is the management of identity and access security that allows people to have control of and manage their digital identities in a secure and decentralized manner. Also, blockchain technology can be used for safe software updates and patch distributions, thus assuring the reliable performance of important system components.
Explainable AI and Trusted AI:
A growing number of AI-based cybersecurity technologies also requires the concept of transparency and trust – the emerging trends. To this end, XAI (Explainable AI) methods are aimed at making AI models more interpretable and comprehensible. In other words, the machine’s security policy officials and authorities could easily determine why the machine made such decisions and recommendations.
The main aim of Trusted AI will be to make AI systems that are fair, precise, and Ethical. This entails a solution to the problems that encompass data privacy, fairness and accountability, therefore, making sure that Artificial Intelligence (AI) controlled security systems are employed with honesty and integrity.
Continuous Monitoring and Adaptive Security:
Cyber threats are diverse and dynamic and require continuous monitoring and flexible security measures. AI-driven cybersecurity products are good in such a mission, which is providing an opportunity to analyze all the incoming data, detecting the abnormalities, and readjusting the defense rapidly. This adaptive security paradigm employs the employment of machine learning algorithms that continuously get information from data and then modify their security policies or controls in response to the new data. Through maintaining the ability to constantly adapt and evolve against the ever-changing threats, organizations can be one step ahead of risk evolving and reducing their vulnerability.
IV. Wrapping Up
In the cyber battlefield where cyber threats lurk, AI-powered cybersecurity solutions are emerging as the ultimate allies for businesses, already providing the advanced abilities to detect, prevent, and mitigate cyber-attacks at an exceptional level of efficiency and accuracy. Through Machine Learning Algorithms and Real-time threat intelligence to data protection and network security, these advanced solutions are redrawing the lines of cybersecurity.
Don’t allow cyber criminals to be one step ahead – unite with Accelerate Tech now and witness the superhero-like security features of AI-based cybersecurity solutions. Get in touch with us for the consultation session and let the experts walk you through the process of integrating the ultimate cybersecurity defense for your business!